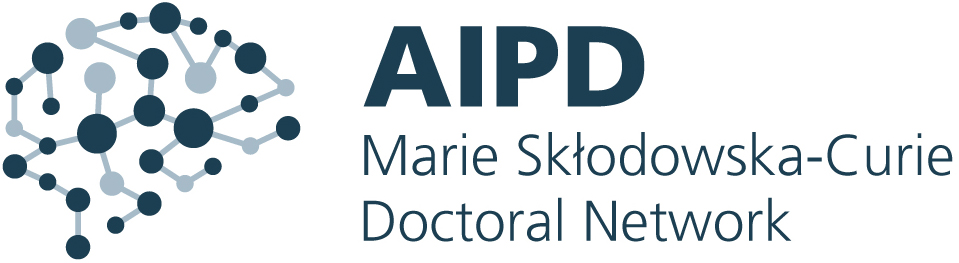
The AIPD Doctoral Network
Artificial Intelligence in Parkinson‘s Disease (AIPD)
We are thrilled to announce 14 PhD open student positions funded by the Marie Skłodowska-Curie Joint Industry-Academic Doctoral Network “AI in Parkinson’s Disease (AIPD)”. AIPD will train a group of 14 Doctoral Candidates in an intersectoral, international and interdisciplinary setting with the aim to educate the next generation of medical data scientists. Our program stands out by a strong translational focus bridging academia and industry. PhD candidates will benefit from:
- Networking Opportunities: AIPD enhances collaboration with leading institutions and researchers in academia and industry across Europe.
- Training and Skills Development: AIPD offers comprehensive training in research and transferable skills, contributing to professional growth.
- Career Advancement: AIPD increases the employability and career prospects of researchers through high-quality research experiences.
- Innovation and Impact: AIPD drives innovation and contributes to societal challenges by supporting cutting-edge research projects in the intersection field of AI and biomedicine.
- Recognition and Prestige: Affiliation with a Marie Skłodowska-Curie program is highly regarded, adding significant value to the researchers’ profiles.
AIPD’s competitive research program covers areas of highest interest for science and society with applications to Parkinson’s Disease:
- Precision medicine
- Digital health
- Trustworthy AI
Find out more about AIPD at https://www.aipd-dn.eu and https://euraxess.ec.europa.eu/jobs
Below are the direct links to the two RCSI-led projects
Project 1
AIPD-DC8-RCSI
Metabolic Blood Biomarkers for Personalised Disease Monitoring
- Host Institution: RCSI University of Medicine & Health Sciences
- PhD Enrolment: RCSI University of Medicine & Health Sciences
- Start Date: October 2025
- Duration: 36 months
- Official PhD Supervisor: Jochen Prehn
Research Objectives
Mitochondrial dysfunction and alterations in energy and lipid metabolism are hallmarks of PD. The aim of this project is to perform a metabolic analysis of blood-based expression profiles to derive clinically accessible, peripheral biomarker signatures based on metabolic dysfunction for personalised PD disease monitoring and targeting.
Specific objectives:
- Identify metabolic signatures in the blood of PD patients and their relation to diagnosis, symptoms and disease progression;
- Predict altered metabolic fluxes/pathways in individual patients by incorporation of gene expression profiles and network-wide analysis;
- Identify metabolic reactions/nodes as putative therapeutic targets;
- Incorporate clinical markers to derive enhanced signatures of metabolic dysfunction for disease monitoring.
The student will first determine differentially expressed metabolites and will perform correlation analyses on metabolomics datasets derived from peripheral blood mononuclear cells (PBMCs) from LuxPARK to describe the heterogeneity of metabolic signatures in PD patients. Signatures/biomarkers will be correlated with diagnosis (IPD, PDD, DLB etc), symptoms (motor, non-motor) and disease stage. Next, at RCSI, the student will be trained in the application of systems biology tools such as genome-scale metabolic models (GSMM) and will generate GSMMs of PBMC/blood gene and/or protein expression profiles to derive functional insight into the distinct metabolic signatures, and predict individual, specifically altered metabolic fluxes/pathways and their relation to diagnosis, symptoms and disease progression. Blood- and brain-based models will be aligned to determine shared and distinct patterns of metabolic dysfunction. Both publicly available datasets as well as brain transcriptomic, proteomic, and metabolomic data generated by the IMI2 PDMitoQUANT project will be available. Knockin/ knock-out and perturbation analyses in silico will predict critical metabolic reactions/nodes and putative therapeutic targets. At UL student will apply AI/ML and XAI techniques to combine clinical features as well as genomic mutations to derive enhanced signatures of metabolic dysfunction to further dissect specific pathways for therapeutic monitoring. Signatures/biomarkers will be validated using PPMI. Together, derived metabolic biomarkers will enable a refined diagnosis of PD patients and allow for longitudinal, personalised monitoring of symptoms. DC8 will closely work with DC14 due to the common focus on metabolic signatures.
Expected Results
- AI/ML models and biomarker signatures for metabolic disease monitoring/staging
- Putative therapeutic targets
Planned Secondment(s)
- Host 1: Centre Hospitalier de Luxembourg
- Duration: 18 months
- Purpose: Learning about PD from a clinical perspective
- Host 2: University of Luxembourg
- Duration: 9 months
- Purpose: Learning about the development of AI/ML models
This project is part of the “Precision Neurology” work package.
https://www.aipd-dn.eu/en/vacancies/AIPD-DC8-RCSI.html
Project 2
AIPD-DC14-RCSI
Identification of Molecular PD Endophenotypes
- Host Institution: RCSI University of Medicine & Health Sciences
- PhD Enrolment: RCSI University of Medicine & Health Sciences
- Start Date: October 2025
- Duration: 36 months
- Official PhD Supervisor: Jochen Prehn
Research Objectives
PD is a heterogeneous neurological condition, and there is significant demand for peripheral biomarkers for patient stratification. The aim of this project is to apply data integration and network inference approaches to derive PD endophenotype signatures for blood-based stratification of PD patients. Specific objectives: 1) Identify multi-modal clusters of co-expressed genes/proteins and gene-regulatory networks associated with disease state; 2) Derive peripheral PD endophenotype signatures; 3) Refine gene signatures for future clinical application. The student will first apply correlationbased network inference approaches on independent multi-‘omic datasets (transcriptome, proteome) from LuxPark and PPMI to identify clusters of co-expressed genes/proteins using unsupervised learning approaches (e.g., MOFA+, clustering, correlation analyses). Data integration approaches incorporating specific genomic markers (e.g., GWAS hits, mutation rates) will then be used to construct multi-modal clusters and infer de novo regulatory networks. Clusters/networks will be correlated with clinical data (disease diagnosis, symptoms, stage) to identify functional clusters associated with particular disease states. Critical regulatory nodes/pathways will be identified by integrating differential expression and network analysis (e.g., centrality). At PETA, the student will apply AI/ML and XAI techniques that can predict an individual’s disease molecular disease endotype. Biomarker signatures will be compared with findings obtained by DR8, i.e. DCs8 and 14 will closely collaborate. DC14 will also collaborate with DC2 due to the common focus on disease stratification (DC2: phenotype level, DC14: molecular level).
Expected Results
- Blood-based endophenotypes of PD
- AI/ML models for patient stratification using blood-based biomarker signatures
Planned Secondment(s)
- Host: Petanux
- Duration: 18 months
- Purpose: Apply AI/ML to predict endophenotype
This project is part of the “Precision Neurology” work package.